Resources
Discover these B2B marketing tips, trends, stories, and actionable guides
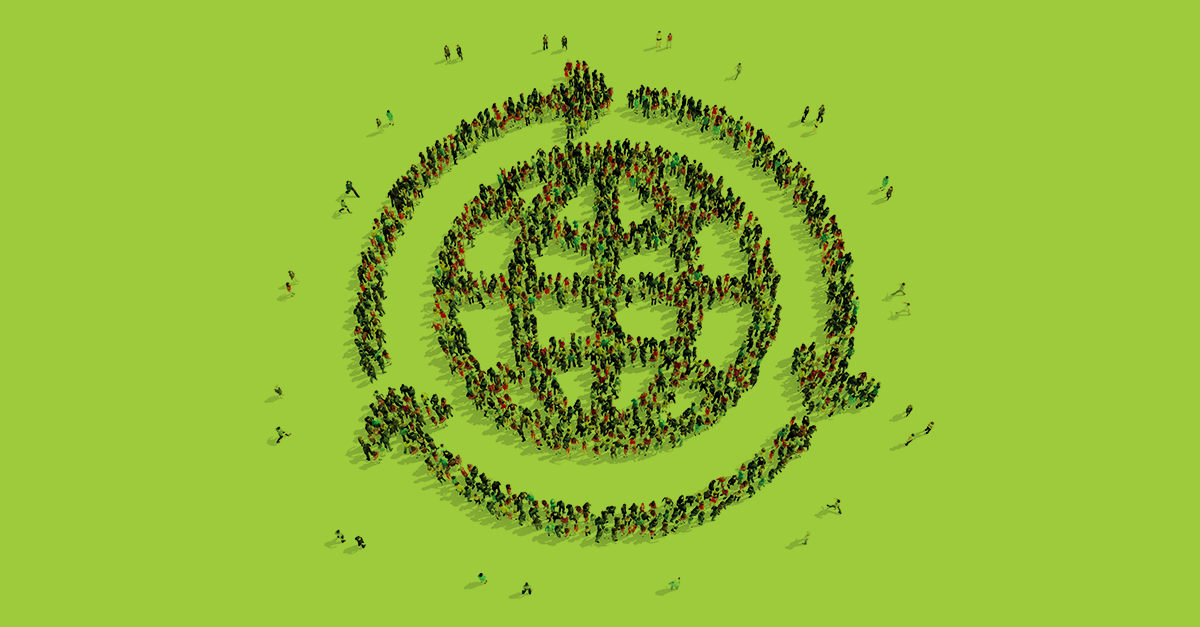
7 reasons content syndication should be a part of your integrated strategy
Content Syndication
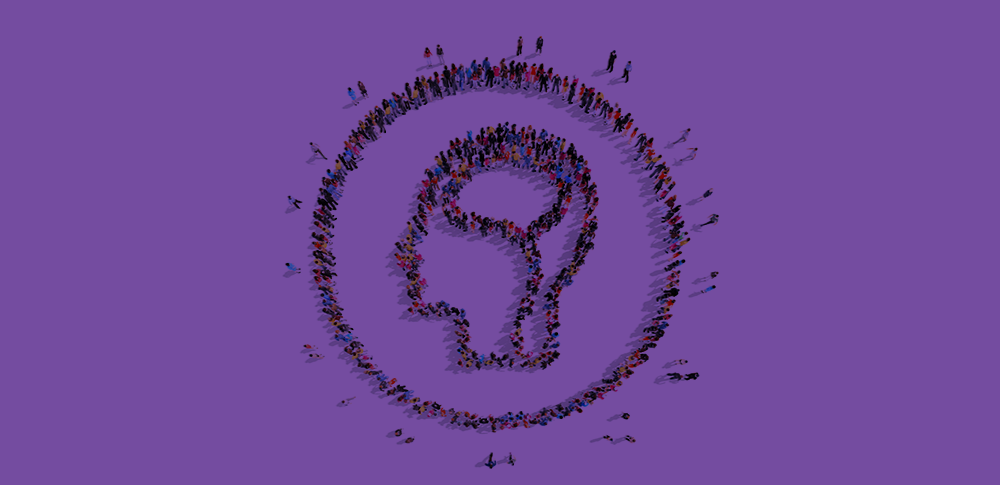
6 takeaways from the 2023 Forrester B2B Summit
Intent Data
Full Funnel Marketing
Buying Groups
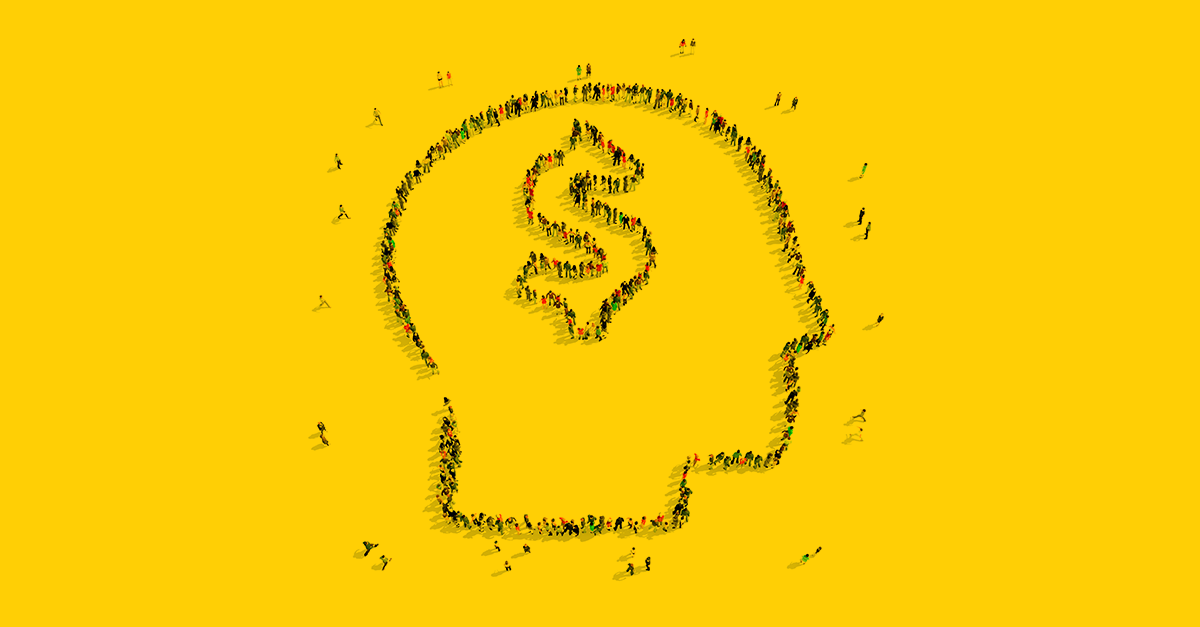
3 Questions to Ask When Setting Your Marketing Budget for 2023
Data Strategy
Industry insights
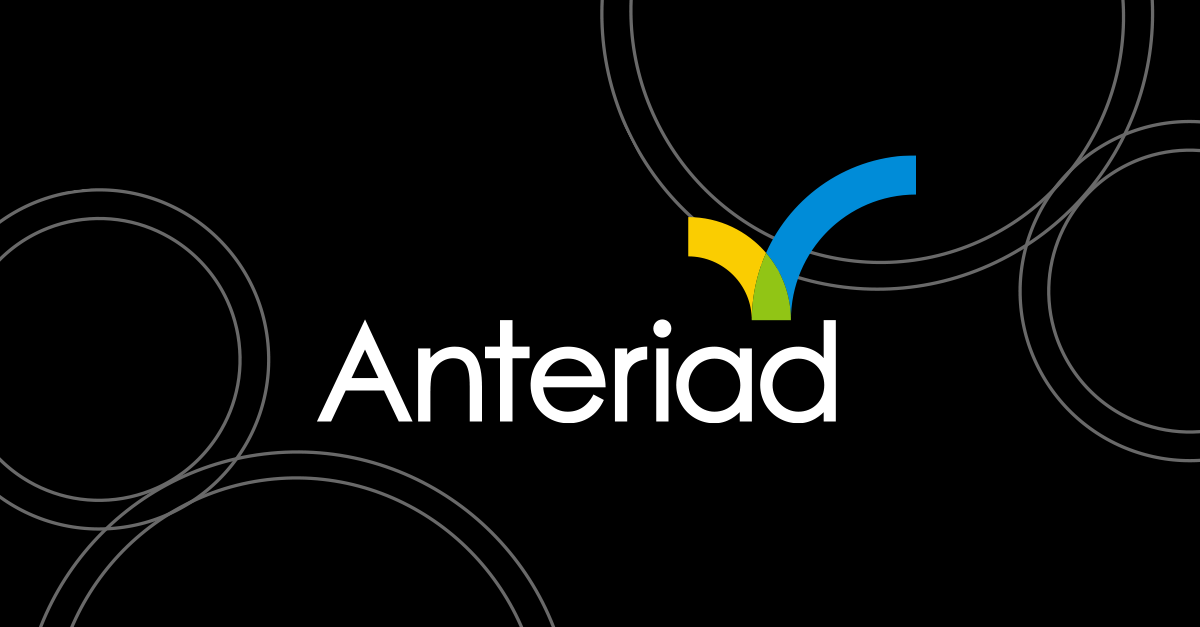
Demand Gen Report: The Importance Of Accuracy, Truth & Transparency In Intent Data
Intent Data
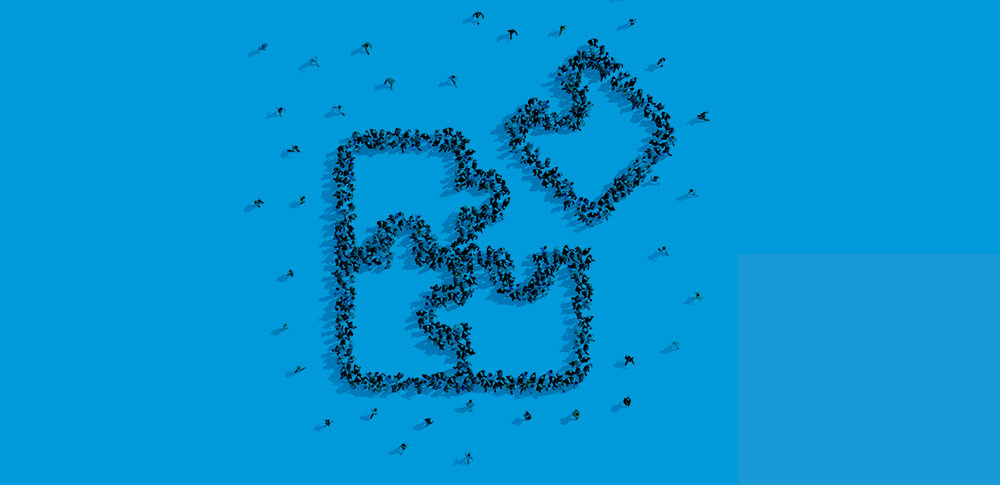
Using Buying Group Intent to Prioritize Your ABM Targets
Account Based Marketing
Buying Groups
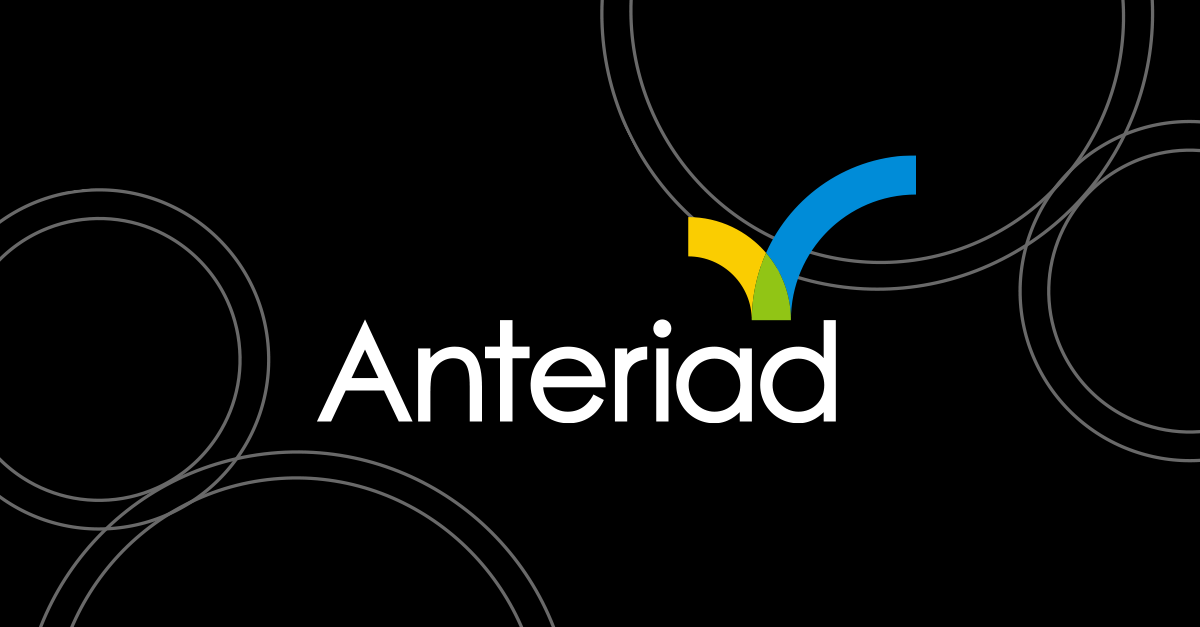
3 ABM Metrics To Make Sense of Today's Data-Driven Marketing Landscape
Account Based Marketing
Sorry, no results found. You can clear the filters or try a different search term.