Optimize reach across channels with our self-service solutions, featuring high-fidelity B2B data and custom audiences.
Resources
Discover these B2B marketing tips, trends, stories, and actionable guides
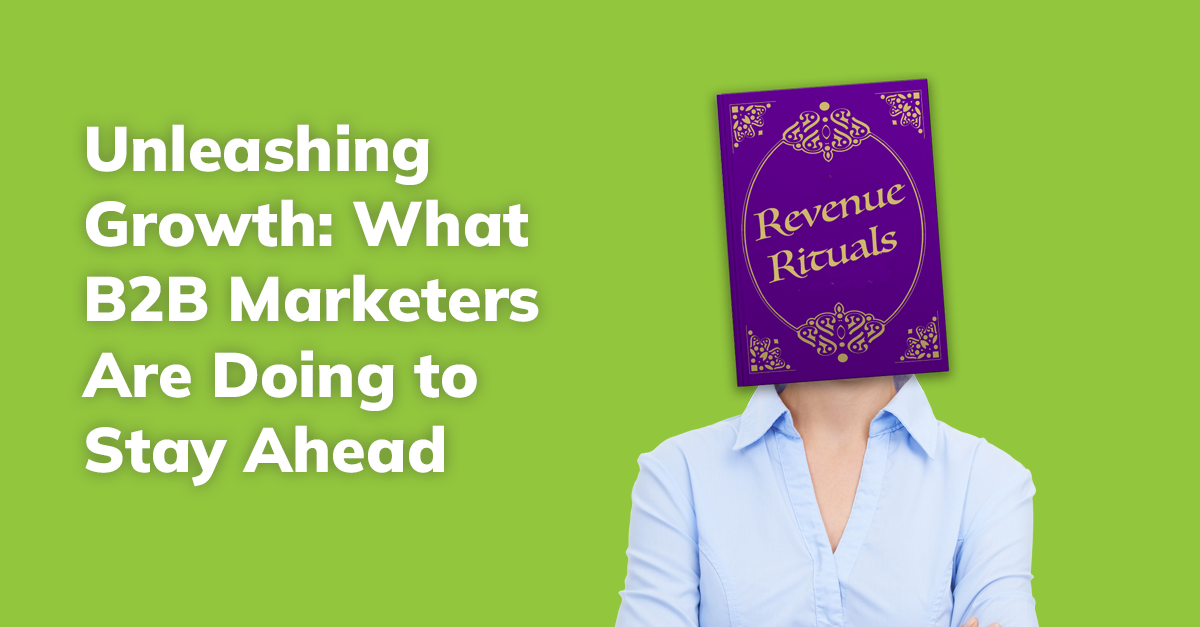
Unleashing Growth: What B2B Marketers Are Doing to Stay Ahead
Industry Insights
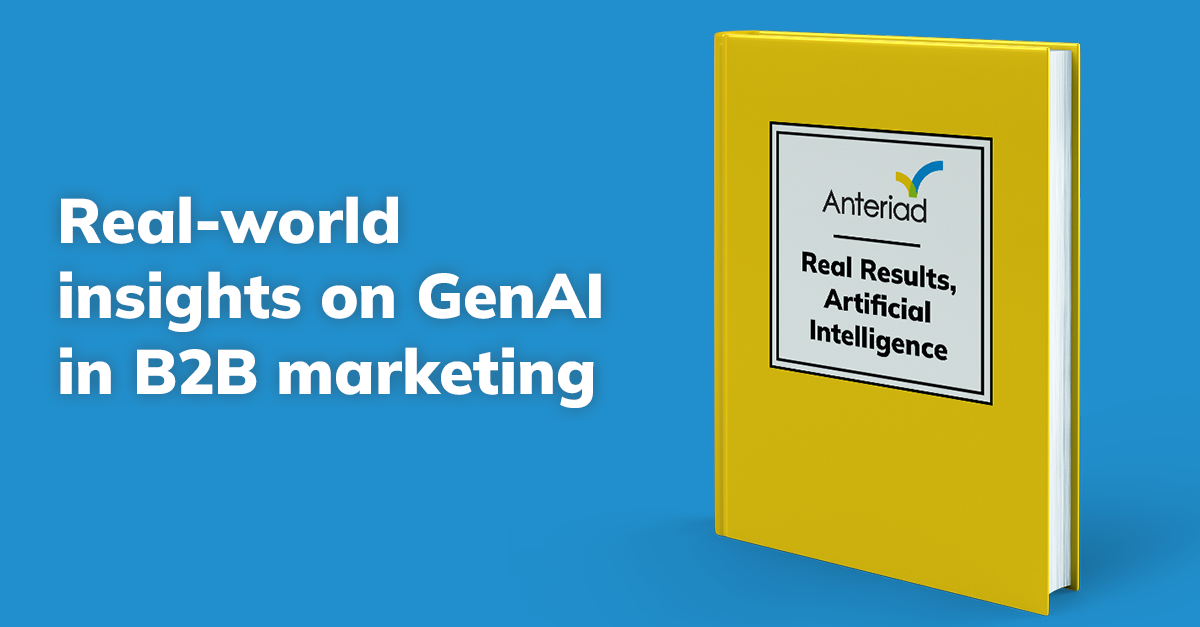
Expert exchange on optimizing B2B marketing with Generative AI
Artificial Intelligence
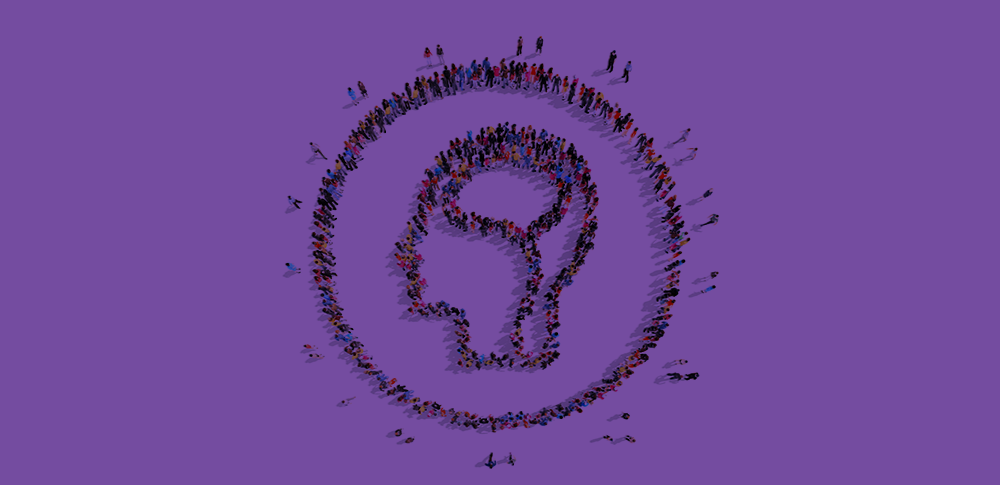
6 takeaways from the 2023 Forrester B2B Summit
Intent Data
Full Funnel Marketing
Buying Groups
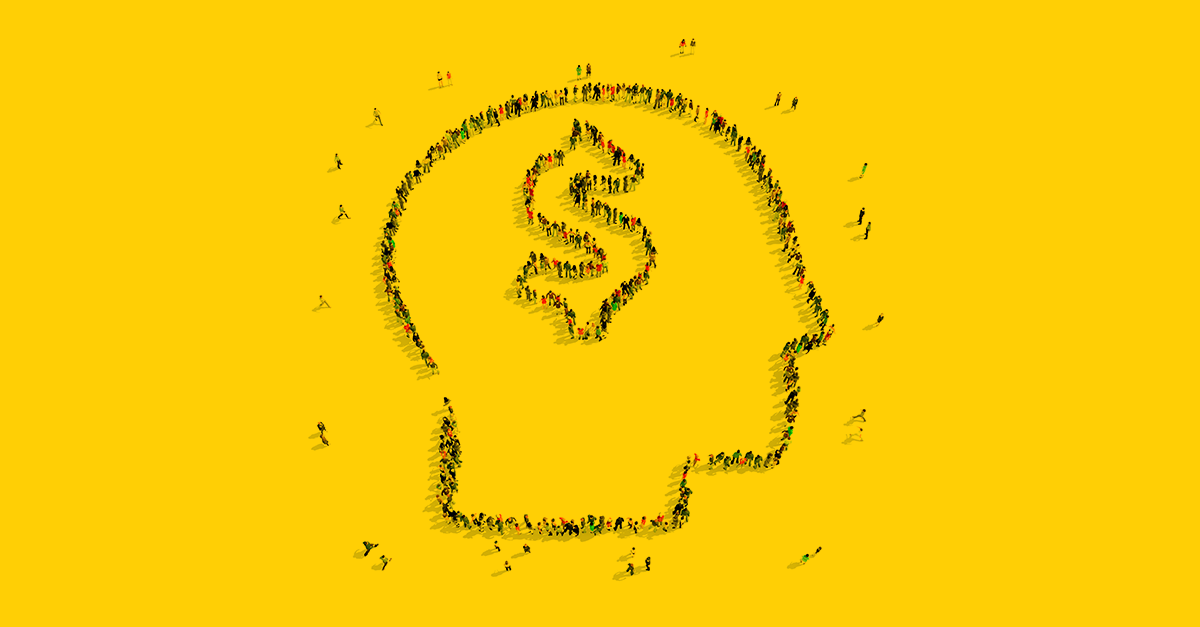
3 Questions to Ask When Setting Your Marketing Budget for 2023
Data Strategy
Industry insights
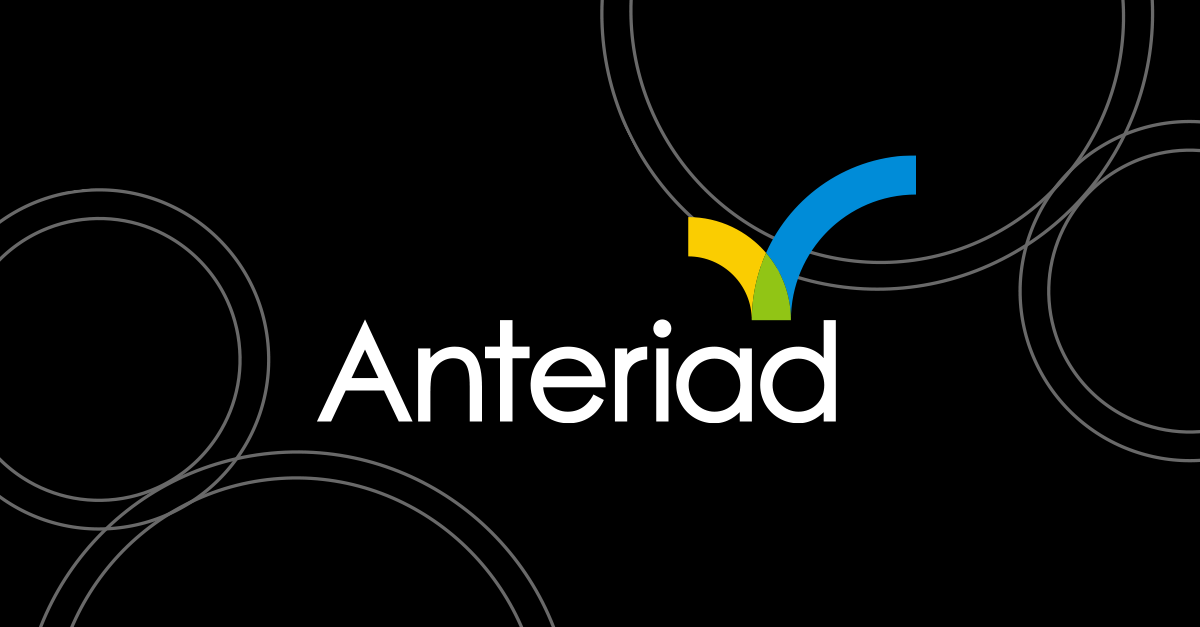
Demand Gen Report: The Importance Of Accuracy, Truth & Transparency In Intent Data
Intent Data
Sorry, no results found. You can clear the filters or try a different search term.